AIot Improves Transparency, Quality for Manufacturers
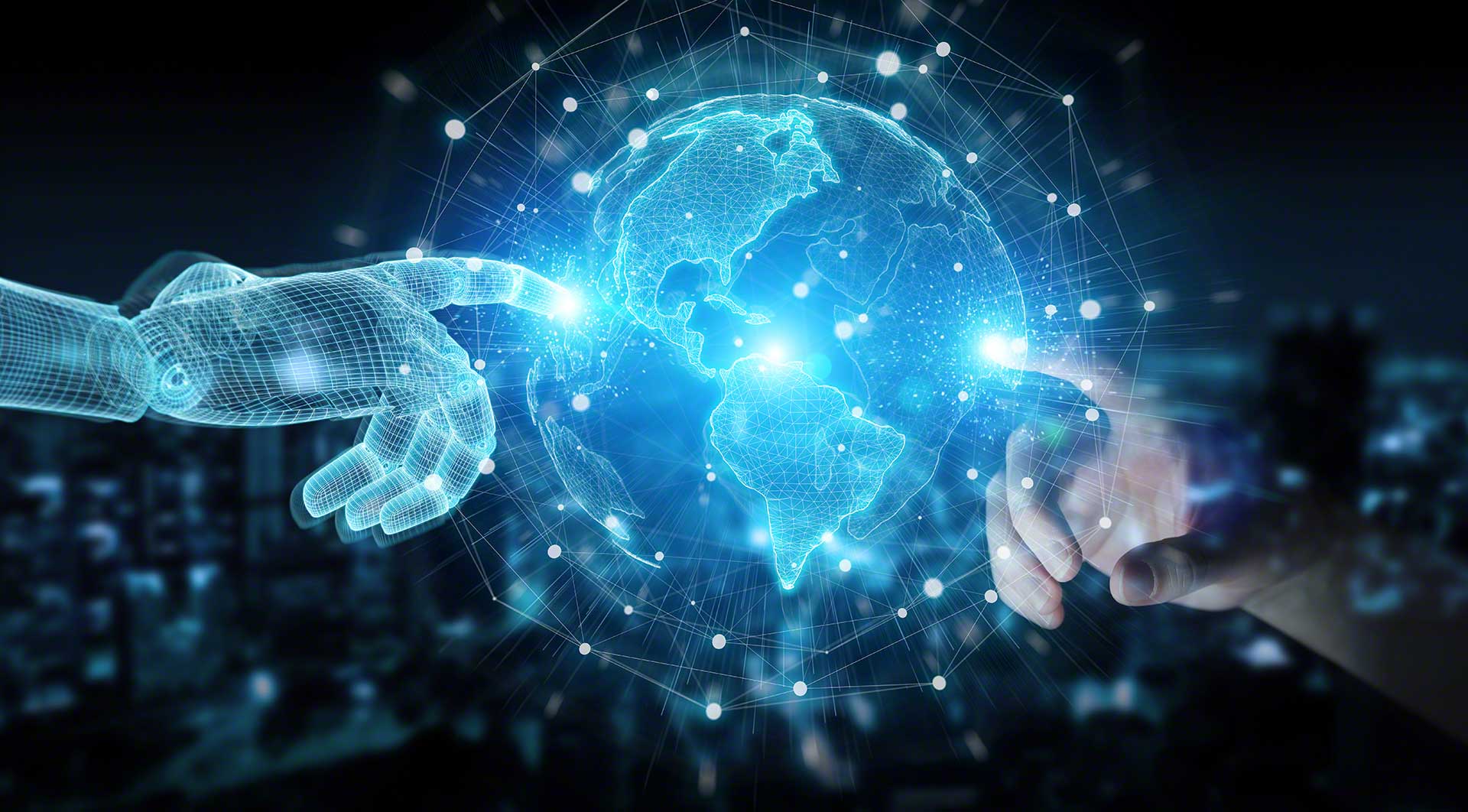
AIot Improves Transparency, Quality for Manufacturers
Plant Engineering Magazine February 2022
By Suzanne Gill
https://www.plantengineering.com/articles/aiot-improves-transparency-quality-for-manufacturers/
The growing use of combined artificial intelligence (AI) and Internet of Things (IoT) solutions across all areas of life has become increasingly noticeable, and this is also true in the industrial sector. At present, in the industrial sector the monitoring of production facilities accounts for the largest share of industrial AIoT applications, according to Martin Plank, corporate research and innovation at Festo.
“AIoT applications can be found in the area of maintenance or quality monitoring, for example in solutions for condition monitoring, predictive maintenance, predictive quality or the management of maintenance activities, for which Festo already offers dedicated solutions with AX and Smartenance,” said Plank. “Increasingly, interesting applications are also being found in the field of energy efficiency and robotics.”
What all these solutions have in common is that they make a contribution to increasing quality, reducing costs and/or maintaining deliverability. In addition to the actual benefits of the solution, organizations are gaining transparency by tapping the data sources required for the applications. The tapped data sources are then often available for the implementation of further applications with significantly reduced effort, resulting in a positive feedback loop.
“However, we have also observed barriers which inhibit the rapid and widespread implementation of new AIoT solutions,” Plank said. “Two key barriers are the quantifiability of the benefits for new solutions and the availability and accessibility of data on machines. Which is especially true for retrofit applications.” There is a clear correlation between these barriers – since an absolute quantification of the benefits of many new solutions is only possible under assumptions, they often need to be evaluated first, for example with a pilot project or a proof-of-concept on a single plant. For the development and evaluation of new solutions, however, data is needed, which can only be obtained with a high effort, especially for existing machines and systems. “For this reason, Festo is working on solutions for simple and flexible data acquisition in production that fill this gap and thus enable the rapid development and evaluation of new customised solutions in the field of AIoT.”
A Powerful Contribution
“Separately, the IoT and AI are making waves in industry,” said Johan Jonzon, CMO and co-founder at Crosser. “However, when combined to form the AIoT the two are capable of delivering intelligent, connecting systems that can self-correct and self-heal themselves.” While the IoT can be likened to a nervous system, giving and receiving signals across a number of devices, AI acts as a brain, receiving data, processing it and using it to make informed decisions.
Until now, IoT technologies – such as cloud computing and storage and machine-to-machine communication – have given manufacturers the ability to connect machines, store data and make it meaningful. Today, with AIoT, manufacturers are presented with more than just facts. Instead of a plant manager only learning about how equipment is performing, and needing to the make their own decisions on how to act upon these findings, AI is closing the loop by automatically taking action.
Also commenting on the barriers to AIoT adoption, Jonzon said: “To make integrating AIoT into a smart factory feasible, manufacturers need a data management system that can support fast decision making. This means analyzing data closer to its source.
“AI activities often need to occur locally to enable fast actions. If the system receives an alert about a machine fault, for example, it needs to act instantaneously to halt production and prevent any serious damage. That’s where the edge comes into play.” By integrating the AI system at the edge, instead of the cloud, latency issues can be avoided, meaning issues such as machine faults can be rectified faster, explained Jonzon.
The same is applicable for process optimization activities, such as changing the speed or type of movement of a machine. An AI system at the edge can send instructions to equipment to improve its performance much quicker than if data was processed only in the cloud, providing a faster route to productivity.
“Another use case where it is advantageous to integrate AIoT at the edge is when visual inspection systems are involved,” Jonzon said. “Cameras and sensors create massive amounts of data, and therefore it makes better sense to analyze and filter this data at the edge, instead of sending such a large mass of information to the cloud.”
Integrating AIoT at the edge can also deliver increased security. Cloud computing can present security issues, as the data is stored by a third-party provider away from the company’s premises, and is accessible over the Internet. Edge computing overcomes these security concerns by filtering out sensitive information at the source and storing it on-premise, so there is less transfer of confidential material to the cloud.
“To successfully integrate AIoT at the edge, it is first necessary to build an AI model offline, then train the model by using previously stored datasets. Once happy with the model, it can then be applied in reality with new live data,” Jonzon said.
“However, applying the model to real time data in an online scenario is very different to testing it on stored data that has already been sorted in the training stage. Real time data hasn’t been filtered or categorized, creating a chaos of information for the AIoT. Therefore, something needs to be done to the data before it can be used by the AIoT.”
The use of edge analytics platforms can prepare the data before it reaches the AIoT. It could, for example, harmonize data that is in different formats, making it possible for the system to interpret.