Revolutionizing Industry with Machine Learning and Deep Learning: Innovations in the Era of Industry 4.0
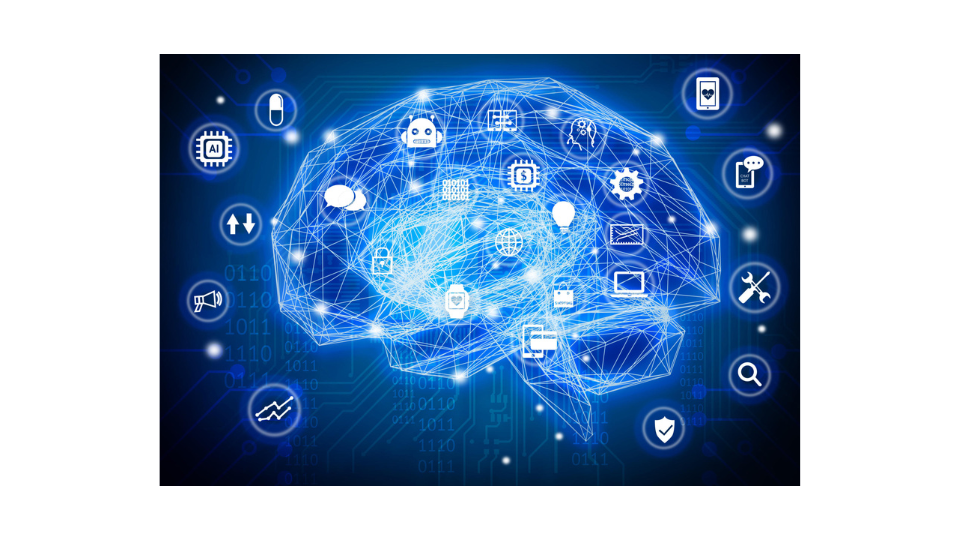
(Source: www.udacity.com)
Revolutionizing Industry with Machine Learning and Deep Learning:
Innovations in the Era of Industry 4.0
By Lydhia Firlanda
As digitalization and Industry 4.0 continue to evolve, the application of artificial intelligence (AI) is becoming increasingly widespread across various industrial sectors. One of the most significant advancements is the use of Machine Learning (ML) and Deep Learning (DL) to enhance efficiency, accuracy, and automation in manufacturing and industrial management. From predictive quality systems to automated production processes, ML and DL are playing a crucial role in shaping the future of smart manufacturing.
This article explores how ML and DL are being implemented in the industrial sector, covering fundamental concepts, key applications, challenges, and future prospects.
Understanding Machine Learning and Deep Learning
Machine Learning (ML) is a branch of AI that enables systems to learn from data and improve performance without explicit programming. Deep Learning (DL), a subfield of ML, utilizes artificial neural networks with multiple layers to analyze and process complex data.
ML and DL algorithms come in various forms, tailored for different industrial needs, including:
- Supervised Learning: The model learns from labeled data to make predictions or classifications.
- Unsupervised Learning: The model identifies patterns in unlabeled data.
- Reinforcement Learning: The model interacts with its environment to optimize decisions based on rewards.
Industrial Applications of Machine Learning and Deep Learning
- Predictive Quality in Manufacturing
One of the most promising applications of ML and DL is predictive quality, which improves product quality by:
- Analyzing sensor data to detect product defects early in the production process.
- Optimizing production parameters to enhance efficiency.
- Predicting potential machine failures to enable proactive maintenance.
- Automated Visual Inspection
Deep Learning, particularly Convolutional Neural Networks (CNNs), has transformed quality inspection by enabling real-time defect detection in products. CNNs can replace manual inspections, which are often slower and prone to human error.
- Predicting and Preventing Production Disruptions
By analyzing historical and real-time data, ML can help industries identify patterns leading to equipment failures or product quality deviations. Long Short-Term Memory (LSTM) networks have been successfully used to predict production issues based on sensor data.
- Supply Chain Optimization
Machine Learning also enhances supply chain management by:
- Forecasting demand.
- Optimizing inventory levels.
- Automating logistics and shipment scheduling.
Challenges in Implementing ML and DL in Industry
Despite their numerous advantages, the adoption of ML and DL in industrial settings comes with several challenges:
- Data Quality and Quantity: High-quality, large-scale datasets are required to ensure accurate model predictions.
- Model Complexity: Deep Learning models can be difficult to interpret and often require significant computational resources.
- Bias and Data Imbalance: If the training data is unbalanced or contains hidden biases, the model may produce skewed results.
- Security and Reliability: AI models used in industrial decision-making must be highly reliable and protected against cyber threats.
The Future of ML and DL in Industry
Machine Learning and Deep Learning have already demonstrated their potential to revolutionize industrial operations. With advancements in Transfer Learning and Continual Learning, AI models are becoming more adaptive and efficient in dynamic production environments.
Looking ahead, we can expect greater adoption of Transformer Networks and Graph Neural Networks (GNNs) to improve data analysis and AI-driven decision-making in industrial settings.
Reference:
- Janiesch, C., Zschech, P., & Heinrich, K. (n.d.). Machine learning and deep learning. https://doi.org/10.1007/s12525-021-00475-2/Published
- Tercan, H., & Meisen, T. (2022). Machine learning and deep learning based predictive quality in manufacturing: a systematic review. In Journal of Intelligent Manufacturing (Vol. 33, Issue 7, pp. 1879–1905). Springer. https://doi.org/10.1007/s10845-022-01963-8